英文原文: http://www.manufacturing.net/articles/2014/02/5-quick-questions-putting-big-data-to-work
中文译文:http://www.infinityqs.cn/Resources/Articles/1287.html
摘要:作为全球统计过程控制(SPC)软件领导者——盈飞无限国际有限公司(InfinityQS International, Inc.)统计方法副总裁,史蒂夫·怀斯(Steve Wise)先生日前接受美国制造业网站(manufacturing.net)专访,就大数据时代数据处理软件的最佳应用实践以及制造企业如何高效利用数据提供的信息这一热门话题展开讨论。
Manufacturing:近期美国制造业网站(Manufacturing.net)发起的一项调查结果显示,约三分之二的被调查者认为自己所在企业并没有对其ERP系统中的数据进行有效利用。虽然这样的声音我们早就听过,并不新鲜,但为什么这种状况长期以来没有得到改善?
盈飞无限国际有限公司 统计方法副总裁 史蒂夫·怀斯
史蒂夫·怀斯:当公司实施一项计划,一切全部落地,数据开始推动企业进行真正的改善行动,毫无疑问将带来巨大的回报。而经常见到的情况是:以数据为基础的系统一旦成功上线,数据开始流动起来并能轻松获取,人们往往就认为项目的实施已经成功。
的确,看到大量数据自动流入数据库感觉非常美妙,但对企业而言,实现数据的可视还只是第一步。根据我的经验,生产数据要真正发挥价值,企业完成以下3个步骤。
首先,处理所有数据流以判断数据的价值。有些数据对现场即时决策有用,有些数据对长期战略决策有用,有些数据则无非是浪费存储空间。实时数据需要通过专业的分析和报警工具在恰当的时间提醒用户采取必要措施或执行指定动作,并且精准发现异常数值,避免用户判断失误,对正常的稳定过程作出不必要的过度反应。
对于用来制订长期决策的数据,系统必须有能力以正确的方式进行分析和处理,确保通过这些数据能够识别出过程中隐含的关键趋势和指标,而没有价值的数据则应该被忽略或另作他用。
其次,ERP系统中的数据仅仅提供了一个数据来源。除此以外,还有质量系统和制造执行系统(MES)中的数据。是否有办法将这些数据有效整合,用于制订企业的商业决策?如果有,怎样才能将这些数据整合到一块?
最后,衡量标准。这些衡量标准能否用于企业自下而上和自上而下的双向决策?这些衡量标准是否足够客观,能够作为公正的奖励依据?
Manufacturing:如果让您说出一个最经常被忽略或最经常被价值低估的数据,您认为是什么数据?为什么?
史蒂夫·怀斯:最经常被忽视的一项数据价值就是:应采用计量型数据或变量数据抽样,而不是计数型数据。大量质量相关数据都被用来测量一些预测价值非常弱的指标,如:产量、放行/待定、合格/不合格、匹配/不匹配等。这些都属于计数型数据,更具体地说,属于不良数据。这些数据可归为1或0(“是”或“否”)的二进制数据。
不良数据只能帮助使用者判断好或者不好。例如:如果产量不好,我们需要的是更具体的计量型数据将好与不好的产品区分开来,并且根据具体的计量型数据去真正解决这个产量不好的问题。计量型数据可进行持续测量,如温度、直径、周期,等。
企业在处理和利用数据时最容易犯的一个错误就是将非常有价值的计量型数据,简单用来判断合格或不合格。要想让数据报表和分析发挥最好的效果,就必须掌握数据的分布,并充分利用其对过程能力的预测价值,而只有计量型数据才能具备这样的价值。
Manufacturing:在盈飞无限最近的一次用户大会上,与会者就有关生产和IT之间的冲突这一话题曾经进行坦诚的对话。针对制造企业中这两个不同部门之间的高效合作,不知您个人有什么建议?
史蒂夫·怀斯:真实的情况是,企业中质量部和IT部有很好的合作基础:他们完全可以非常轻松地让对方的业绩都非常漂亮,实现双赢。当前制造企业内部的各种系统越来越复杂,导致大量信息真空;所以,当质量平台能够将针对性的将智能信息传递给最能利用其价值的人时,企业将大大从中受益。质量部和IT部的一个共同诉求是:摆脱依靠“拍脑袋”做决定或单凭经验做判断的落后决策模式,因此,当企业形成以数据为导向和依据的管理文化,这两个部门都将直接受益。
尽管如此,其实很多非常成功的软件应用项目都是由IT部门主导的。而其中最成功案例往往都有一个共同秘诀:IT部的项目负责人切实将生产使用部门的需求作为项目的指导方向。生产与IT间的矛盾被放大往往是因为IT部介入项目的时间太晚,此时往往给人的印象是生产部门希望绕开IT来做这个项目,在想尽办法避免“IT造成的不必要延迟”。结果,当非IT人员终于意识到他们的技术短板时,两个部门间的裂痕已然产生。
Manufacturing:如果请您给美国制造企业一样东西,您会给什么?为什么?
史蒂夫·怀斯:从数据管理这个层面来说,我会鼓励制造企业针对我们收集的每一条数据,自己回答以下这几个最基础的问题,确定收集这些数据的目的究竟是什么。
§ 这些数据服务的客户是谁?
§ 需要根据这条数据做出什么决策或判断?
§ 当数据值开始上升(或下降或保持不变),这是好事还是坏事?
§ 当这些数据显示需要采取某些行动,企业是否已经有相应的机制对这些数据作出快速响应?
通过回答上述问题,企业就能判断究竟哪些数据可用来做出实时判断、哪些数据最好用来做长期战略决策、应该采取的纠正行动是什么,以及哪些数据根本就没有用,无非浪费空间而已。
英文原文:
A recentManufacturing.netsurveyfound that nearly two-thirds of respondents felt their organization wasn’teffectively using all the data housed in their ERP system. This was neithersurprising nor new, but why does this trend continue?
I can’t tell you how rewarding it iswhen a company implements a plan and the light bulb goes on and they finallysee how data can drive truly effective action. When data-based systems areinstalled, the implementation is too often considered successful once the dataare flowing and people can get to the data.
Yes, watching data automatically flowinto a database is a beautiful experience,but visualizing data is justthe first step. There are three milestones I look for in order to mosteffectively put manufacturing data to work.
The first is toprocess all thestreams of data to determine their usefulness. Some data are useful for makingreal-time decisions, some data are useful for making long-term strategicdecisions, and some data are just taking up space. Real-time data need specialanalysis and alerting tools to warn you if a change is needed and also toprovide feedback that indicates true outliers so you don’t change anything thatscrews up a stable situation.
Data that are used for longer-termdecisions need to be cobbled together in a way that exposes all key trends andindicators, while useless data need to be turned off, throttled back orrepurposed.
Second,data in an ERP system areonly one source. What about data from Quality and Manufacturing Executionsystems? Are there meaningful ways to integrate that data to aid in decisionmaking? If so, what needs to happen to bring those data together?
And lastly, what about the metrics? Dothey support decision-making both up stream and down? Do they reward the rightbehavior?
If you had toidentify one data set that is often over-looked or under-utilized, what is itand why do you think that’s the case?
The most overlooked data opportunity isto use variables data sampling rather than attribute. Many quality-related datastreams are measuring weak indicators such as yield, go/no-go, pass/fail, orconforming/nonconforming. These types of data are all attribute data; morespecifically, defectives data. These data boil down to a 1 or a 0.
Defectives data sets only help someone feelgood or feel bad about whatever those data represent. If the yield is bad, morepowerful variables data are needed to isolate and help solve the problems.Variables data can be measured on a continuous scale, such as temperature,diameter and cycle time.
One of the biggest mistakes companiesmake with industrial data is to take a stream of variables data and dumb itdown to a pass or fail. A better way to report and visualize data is tounderstand the data set's distribution and predictability. This is efficientlyachieved only with variables type data.
At the recentInfinityQS user’s conference, some light-hearted remarks were made about theconflicts between operations and IT. What advice would you offer in helpingthese two areas of manufacturing to work together more effectively?
The truth is that Quality and IT have anopportunity to make each other look very good. The complexities of today’smanufacturing systems result in so many visibility gaps that it becomes a hugewin when a quality platform is able to deliver relevant intelligence to thepeople that can most effectively use it. Quality and IT both have an interestin taking the guesswork out of manufacturing operations and both greatlybenefit when a company’s culture becomes more data driven.
That said, many of the most successfulsoftware-based projects are led by the IT department. The most successful ofthose are when the IT leaders rely on Operation's user requirements as theirguide. The problems between Operations and IT are magnified when IT is broughtinto a project late that was ill conceived by someone in Operations who triedto bypass IT and just get the project done without "unnecessary ITdelays." Eventually, non-IT folks realize their technical shortcomings,but the damage is already done.
If you couldgive U.S. manufacturers one thing, what would it be and why?
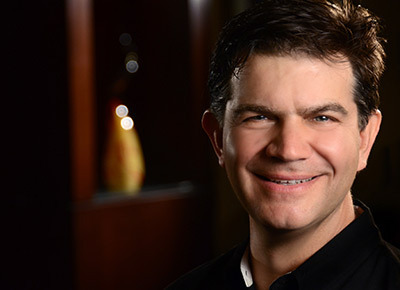
When it comes to data management, Iwould encourage manufacturers to ask basic questions that challenge the purposeof every stream of data.
§ Who is the customer of the data?
§ What decisions are going to be made withthat data stream?
§ If the data values start to increase (ordecrease or remain the same), is that good or bad?
§ If the data stream says that somethingneeds to be done, is there any infrastructure in place to act on the data?
The responses to these questions willhelp identify what data are needed for real-time decisions, what data are bestused for long-term strategic decisions, what the corrective actions are andwhat data just might become a waste to collect.